2024’s top marketing trends revealed—how FADS shaped us and what’s next for 2025, from AI to rebranded vices.
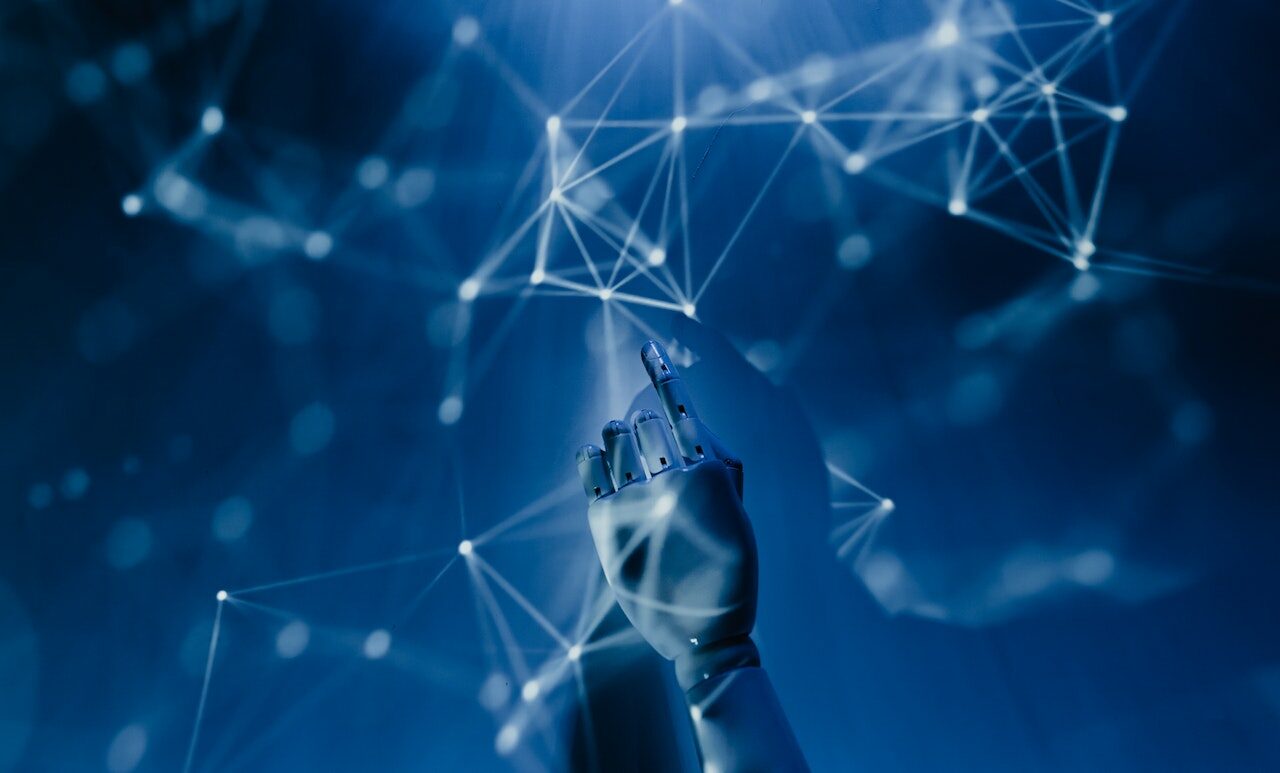
AI in Drug Development: Revolutionizing the Pharmaceutical Industry
Artificial Intelligence is a game changer
We hear a lot about artificial intelligence (AI) lately, thanks in part to the rise in popularity of ChatGPT and Bard. Machine learning has been making waves in various industries, from finance and marketing to robotics and manufacturing. But one area that caught our attention recently is the use of AI in drug development. With AI, the process of bringing new drugs to market could be revolutionized. Let’s dive down this rabbit hole together and explore what’s going on.
AI can crunch massive data sets
The drug development process is a long, complex journey with multiple stages, from target identification and drug discovery to clinical trials and regulatory approval. The use of AI in drug development has the potential to speed up the process, reduce costs, and improve the success rate of drug development.
With the ability to analyze large amounts of data from various sources, scientists can build better models to identify potential drug formulas for more effective and safer drugs. But what does that mean?
Here’s an example. Over the past decade, there have been some big initiatives aimed at gathering massive amounts of cancer data. This data comes from looking at tumor samples and can be used to help us figure out what might work when it comes to treating cancer.
All of this data is pretty awesome, but it can be overwhelming too. That’s where the AI integration part comes in. Instead of looking at just one type of data, scientists can review lots of different types at the same time. And when they do that, they start to see patterns they might not have noticed otherwise. So, there you have it. By gathering all this data and crunching it with AI, researchers are starting to make some serious headway in the fight against cancer.
AI can help guide clinical trial decisions
Better models built with available data can also lead to improvements in clinical trial design and recruitment too. Clinical trials are an essential part of drug development, but they can be expensive, time-consuming, and often have low success rates.
Clinical trials typically evaluate a drug’s effectiveness by looking at the average effect it has on a group of people. They count the number of folks who see improvement versus those who don’t. If there’s enough improvement, the drug is considered successful; if not, it’s a bust. However, this approach can sometimes disregard smaller groups of people who actually benefited from the drug.
As an example, AI was able to predict which patients might best respond to Trastuzumab Deruxtecan, allowing those with the best chance of response to be in the clinical trial, and potentially saving those who the drug was not likely to work on from experiencing unnecessary side effects. And in some cases, these patients can try other drugs that may work for them, saving time and lives.
“Patients can have this terrible experience of going in and out of hospital, sometimes for years, getting drugs that don’t work.” said Richard Law, chief business officer of Exscientia to the MIT Techonology Review.
AI can guide messaging and sales
The pharmaceutical industry has always had loads of data, especially in the US. In recent years, pharmaceutical companies have gotten better at collecting data from internal and external sources, and they’re doing a better job of making sure that data is accurate and useful. But when it comes to analyzing that data, they’re still pretty limited.
Enter AI: as competition heats up, pharmaceutical companies are harnessing AI to make sense of all that data to make predictions and recommendations. It’s a big step forward for the industry, and it could help them get even better at personalizing their messaging and improving sales.
In fact, they are already working on how to boost pharmaceutical sales and marketing with artificial intelligence as outlined in this whitepaper.
Companies that want to really hit it big with customers will hop on the AI train and personalize their approach, keep themselves in the spotlight, and make sure their promotional channels are all singing the same tune. It’s not just about pushing the clinical benefits of a product anymore – savvy sales and marketing teams who use AI to create a seamless customer experience will stand out, gain more customers, and make it rain with a higher sales impact.
But wait, we’ve still got some challenges
While the use of AI in drug development brings significant benefits, there are also challenges and potential drawbacks that need to be considered and addressed.
Data quality and availability
AI requires large amounts of high-quality data to be effective. However, much of the data in drug development is fragmented, unstructured, or of poor quality, which can affect the accuracy and reliability of AI predictions.
Suggestions to overcome this challenge include standardizing data collection and sharing, as well as investing in advanced technologies like natural language processing and data mining to pull valuable information from unstructured data sources. Collaboration and data sharing between industry stakeholders are additional ways to expand the pool of available data and improve its quality.
Interpreting and explaining results
AI models can be complex and difficult to interpret, which makes it challenging for researchers to understand how the predictions were made. This lack of transparency makes it difficult to validate the predictions and ensure they’re unbiased. To combat this, teams are developing transparent AI models with clearly defined inputs, outputs, and decision-making processes. The goal is to help researchers better understand how AI models make predictions and recommendations.
Regulatory and ethical considerations
The use of AI in drug development raises regulatory and ethical considerations, such as the need for transparency, privacy, and fairness. There is also a risk that AI could perpetuate or exacerbate existing biases in drug development, which could have negative consequences for patient outcomes. With these considerations in mind, the industry should engage with regulators and stakeholders early in the development process to ensure compliance with regulations and ethical standards. Investing in multi-disciplinary teams, with experts from diverse fields such as medicine, ethics, law, and computer science can also help address regulatory and ethical concerns.
The exploration has just begun
“Bringing [computer science and biology] together creates so many opportunities for change in, not just in drug discovery, but in agriculture technology, in environmental technology, in energy, and in biomaterials. I think there’s just a tremendous wealth of directions that one can take those fields and bring them together in interesting ways.” – Daphne Koller, No Priors Podcast
The use of AI in drug development has the potential to revolutionize the pharmaceutical industry by making drug development faster, more efficient, and more cost-effective. As researchers and companies continue to harness the power of AI in drug development, expect to see new breakthroughs in medicine, and other fields, that will improve the health and well-being of people around the world.
They've got their hooks in you.
FADS rise quickly, burn hot and fall out. They say you're fat, you're no fun, you need to relax, and you might even die alone.
In fact, FADS bank on the fact that you already believe all of that.
Ready to learn how it works?